Research Article - Der Pharma Chemica ( 2021) Volume 13, Issue 5
Quantitative Structure-Activity Relationship and Molecular Modeling Studies on a series of chiral tetrahydronaphthalene-fused spirooxindole as CDK4 inhibitor against Glioblastoma
Anjali Sharma and Neeraj Agarwal*Neeraj Agarwal, Department of Biotechnology, Meerut Institute of Engineering and Technology, Meerut 250005, India, Email: neeraj.agarwal@miet.ac.in
Received: 10-May-2021 Accepted Date: May 23, 2021 ; Published: 28-May-2021
Abstract
Cyclin Dependent Kinase 4(CDK4) is responsible for the progression of cell cycle at G1-S phase. CDK inhibitor alters the cell cycle that will inhibit cancer cell formation. Spirooxindole derivative targets p53-MDM2 interaction that inhibits CDK4 protein which results in inhibition of cell cycle. Spirooxindole is a unique three- dimensional scaffold that was first isolated from Rubiaceae and Apocynaceae plants. Spriooxindole is a promising drug through many years which can be used to treat an array of diseases such as cancer, analgesic, mental disorder, bacterial and viral infection. From past decade spirooxindole became a huge topic of interest in bio medicinal field due to its multipotentability. In our present work, we have performed QSAR (Quantitative Structure-Activity Relationship) and Molecular modeling studies on a series of chiral tetrahydronaphthalene-fused spirooxindole, resulting in a cross validated r2cv value of 0.858 obtained by Leave one out method (LOO), and predicted r2pred.0.622 with coefficient of correlation of (r) that was obtained by the multiple regression analysis is0.949.A series of new compounds were designed based upon the generated QSAR model. Afterwards these compounds were docked with the protein and checked for their ADME properties. Toxicity of the new compounds was also predicted.
Keywords
CDK4, Spirooxindole, QSAR, Molecular Docking, ADME, Toxicity
Introduction
Cyclin Dependent Kinase 4 is a Kinase holoenzyme that possess 2 subunit- a cyclin that is regulatory subunit and a catalytic subunit. CDK4 is Thr172, and is associated with D-type Cyclin in mammalian cells and mediates cell cycle progression through G1 phase [1]. CDK4-Cyclin D complex activation contributes to hyper phosphorylation of the Rentinoblastoma (Rb) protein. CDK4-Cyclin D complexes possess a non-catalytic function, whereas its association with p21 in G1 phase seize the CKIs and allows the cell progression. p21 also inhibits the CDK4 complex under certain growth conditions [2,3]. p53 regulates the transcription of p21 and p21 inhibits Cdk2 that leads to arrest of G1 upon DNA damage [4,5]. According to the various studies CDK4 and Cyclin D1 over expresses in various types of tumor [6-10], which leads to hyper activation of CDK4 in a vast variety of tumors. Under certain growth conditions p21 inhibits the CDK4-Cyclin D complexes [2,3,11]. So, it has been suggested that inhibitors of this kinase will be an efficient therapeutic agent to treat cancer that proliferates due to CDK4 [12].
Spirooxindole is a natural product which belongs to the family oxindole alkaloids [13] and it was first isolated from the Rubiaceae and Apocynaceae plants. Spirooxindole is a unique three-dimensional scaffold that possess atleast 3 heterocyclic systems. According to previous studies Spirooxindole and its derivatives can be used as multi therapeutic drug to treat an array of diseases such as Cancer, Malaria, Inflammation, viral & microbial infection, diabetes [14].Mechanism of spirooxindole derivatives targeting p53-MDM2 interaction were the main focus of scientists in previous years. Spirooxindole is basically a five membered heterocyclic and carbocyclic ring that contains oxindole at C-3 position. Huang et al. synthesized a series of spiropentenoneoxindoles by using C-Piancatelli rearrangement reaction and checked for their anti-proliferating activity in tumor cell lines [15].
Zohu & group synthesized five-membered carbocyclic spirooxindoles series complexed with substituted oxindoles which were checked for their antitumor activity in leukemia, lung cancer and prostate cancer cell lines [16].
Arumugam et al. synthesized a series of Spiroheterocycles containing pyrrolidine, indole/imidazole, and spirooxindole. Studies shows of this series shows that these compounds could inhibit epithelial cell line by apoptotic cell death [17]. Syamsivappan et al. synthesized a series of pyrrolidine 8- nitroquinolone containing spirooxindole that possess remarkable potency against cervical cancer cell line [18,19].
Quantitative structure–activity relationships (QSARs) aims to correlate chemical structure to biological activity through statistical approach [20]. QSAR models are extensively used in both drug discovery and environmental toxicology [21] to check the activity of untested chemicals. Wang et al. synthesized a series of chiral spirocyclictetrahydronaphthalene (THN)- fused spirooxindole and the compounds were checked for their dual inhibitory effect on MDM2-CDK4 against glioblastoma cell line [19]. In this paper we have prepared a QSAR model, On the basis of that model we have reported some new compounds that may have higher activity and less toxicity. The ADME/T properties of these compounds have also been studied and checked. Also we checked their hepatotoxicity, carcinogenecity, mutagenecity, cytotoxicity and Heat shock factor response gelement (HSE) with the help of ProTox-ll and admetsar2 (Figure 1).
Materials and Methods
We took a series of chiral spirocyclictetrahydronaphthalene (THN) - fused spirooxindole that possess forty molecules. These molecules act as CDK4 inhibitor and checked for their antitumor activity [19]. All these compounds are listed in Table 1 along with some Weighted Holistic Invariant Molecular Descriptors that governs the potency of these compounds. The most significant parameters governing their potency were found to be Ks (K global shape Index/Weighted by related I-state), E3e (3rdcomponent accessibility directional WHIM index/weighted by relative Sanderson electro negativities), P2u (2nd component shape directional WHIM index/Unweighted). All these parameters were calculated by Chem Des, a free online tool to calculate molecular descriptors and have almost negligible mutual correlation (as shown in Tables 2 & 3). Protein Data Bank was used to take out protein (PDB ID 2W9Z) to perform Docking studies and to examine the interactions between the predicted compounds by employing Molegro Virtual Docker software and a new series of 10 compounds was designed by the help of Swiss ADME. The ADME/T properties were predicted by using Swiss ADME and admetsar2, which calculates the molecular weight, H-bond donor and H-bond acceptor counts, octanol-water partition coefficient (log ), polar surface area, and bioavailability (optional) of the molecules and verifies Lipinski, Ghose, Veber, Egan and Muegge rule. It also shows GI absorption of molecule and availability of P-gp substrate. Afterwards, toxicity of predicted compounds was checked with the help of Pro Tox-ll, [22] where Hepatotoxicity, Carcinogenicity, Mutagenicity, Cytotoxicity and Heat shock factor response element (HSE) were checked (Figure 2).
![]() |
||||||||||||
---|---|---|---|---|---|---|---|---|---|---|---|---|
S.No. | R1 | R2 | Ks | E3e | P2u | log(1/IC50) | ||||||
obsd | Calculated | Pred (LOO) | ||||||||||
1 | H | ![]() |
0.48 | 0.34 | 0.27 | 4.37 | 4.32 | 4.31 | ||||
2 | H | ![]() |
0.36 | 0.34 | 0.28 | 5.16 | 5.07 | 5.06 | ||||
3 | 5-F | ![]() |
0.48 | 0.35 | 0.26 | 4.27 | 4.28 | 4.28 | ||||
4c | 5-F | ![]() |
0.35 | 0.37 | 0.28 | 5.59 | 5.23 | - | ||||
5 | 7-F | ![]() |
0.48 | 0.41 | 0.26 | 4.28 | 4.48 | 4.50 | ||||
6 | 7-F | ![]() |
0.35 | 0.35 | 0.29 | 5.59 | 5.23 | 5.18 | ||||
7c | 5-Cl | ![]() |
0.49 | 0.38 | 0.26 | 4.15 | 4.33 | - | ||||
8 | 5-Cl | ![]() |
0.35 | 0.34 | 0.28 | 5.28 | 5.13 | 5.11 | ||||
9 | 6-Cl | ![]() |
0.49 | 0.34 | 0.26 | 4.21 | 4.19 | 4.19 | ||||
10c | 6-Cl | ![]() |
0.35 | 0.36 | 0.28 | 5.07 | 5.19 | - | ||||
11 | 4-Br | ![]() |
0.49 | 0.37 | 0.26 | 4.34 | 4.29 | 4.29 | ||||
12 | 4-Br | ![]() |
0.36 | 0.35 | 0.28 | 5.03 | 5.10 | 5.11 | ||||
13 | 5-Br | ![]() |
0.49 | 0.35 | 0.26 | 4.12 | 4.23 | 4.23 | ||||
14c | 5-Br | ![]() |
0.35 | 0.32 | 0.28 | 5.39 | 5.06 | - | ||||
15 | 6-Br | ![]() |
0.49 | 0.38 | 0.26 | 4.23 | 4.33 | 4.33 | ||||
16c | 6-Br | ![]() |
0.35 | 0.34 | 0.28 | 5.54 | 5.13 | - | ||||
17 | 5-NO2 | ![]() |
0.49 | 0.37 | 0.24 | 4.37 | 4.16 | 4.14 | ||||
18 | 5-NO2 | ![]() |
0.30 | 0.37 | 0.27 | 5.10 | 5.44 | 5.53 | ||||
19c | 5-Me | ![]() |
0.44 | 0.31 | 0.28 | 4.17 | 4.51 | - | ||||
20 | 5-Me | ![]() |
0.30 | 0.33 | 0.26 | 5.15 | 5.24 | 5.28 | ||||
21 | H | ![]() |
0.46 | 0.36 | 0.27 | 4.40 | 4.50 | 4.50 | ||||
22 | H | ![]() |
0.41 | 0.46 | 0.25 | 4.51 | 4.98 | 5.07 | ||||
23 | H | ![]() |
0.47 | 0.37 | 0.27 | 4.41 | 4.47 | 4.48 | ||||
24 | H | ![]() |
0.41 | 0.47 | 0.25 | 5.03 | 5.02 | 5.01 | ||||
25 | H | ![]() |
0.46 | 0.37 | 0.27 | 4.32 | 4.53 | 4.54 | ||||
26 | H | ![]() |
0.41 | 0.48 | 0.25 | 5.49 | 5.05 | 4.95 | ||||
27 | H | ![]() |
0.47 | 0.37 | 0.27 | 4.45 | 4.47 | 4.47 | ||||
28 | H | ![]() |
0.41 | 0.44 | 0.24 | 5.07 | 4.85 | 4.80 | ||||
29 | H | ![]() |
0.47 | 0.37 | 0.27 | 4.53 | 4..47 | 4.47 | ||||
30 | H | ![]() |
0.38 | 0.46 | 0.27 | 5.07 | 5.29 | 5.31 | ||||
31c | H | ![]() |
0.52 | 0.36 | 0.25 | 4.41 | 4.02 | - | ||||
32c | H | ![]() |
0.47 | 0.51 | 0.22 | 5.08 | 4.61 | - | ||||
S.No. | R1 | Ks | E3e | P2u | log(1/IC50) | |||||||
Obsd | Calculated | Pred (LOO) | ||||||||||
33b | 5-Cl | 0.49 | 0.38 | 0.26 | 6.11 | 4.33 | - | |||||
34 | 5-Cl | 0.35 | 0.34 | 0.28 | 5.41 | 5.12 | 5.09 | |||||
35 | 5-Cl | 0.29 | 0.51 | 0.35 | 6.31 | 6.50 | 6.63 | |||||
36 | 5-Cl | 0.41 | 0.50 | 0.29 | 5.59 | 5.38 | 5.35 | |||||
37 | 5-Br | 0.29 | 0.51 | 0.35 | 6.60 | 6.50 | 6.44 | |||||
38 | 5-Br | 0.41 | 0.50 | 0.29 | 5.43 | 5.38 | 5.38 | |||||
39b | 5-Br | 0.49 | 0.35 | 0.26 | 6.49 | 4.23 | - | |||||
40 | 5-Br | 0.35 | 0.32 | 0.28 | 4.97 | 5.06 | 5.07 |
S.No. | Predicted Compounds | Ks | E3e | P2u | log(1/IC50) |
---|---|---|---|---|---|
1 | ![]() |
0.24 | 0.48 | 0.41 | 7.09 |
2 | ![]() |
0.23 | 0.47 | 0.40 | 7.05 |
3 | ![]() |
0.24 | 0.48 | 0.40 | 7.02 |
4 | ![]() |
0.21 | 0.45 | 0.40 | 7.09 |
5 | ![]() |
0.23 | 0.45 | 0.39 | 6.91 |
6 | ![]() |
0.27 | 0.49 | 0.41 | 6.95 |
7 | ![]() |
0.26 | 0.48 | 0.41 | 6.98 |
8 | ![]() |
0.27 | 0.48 | 0.41 | 6.92 |
9 | ![]() |
0.27 | 0.48 | 0.39 | 6.79 |
10 | ![]() |
0.26 | 0.47 | 0.41 | 6.94 |
Results and Discussions
QSAR Results
All the compounds of Table 1 were divided into two subsets: training set and test set. For test set, the compounds were selected randomly by keeping in mind the wide variation in their structures and a good span in their activity data. Compounds with superscript ‘c’ were taken for test set and rest all the compounds were taken in training set except the compounds with superscript ‘b’, those were taken as outliers in Table 1. A multiple regression analysis (Hansch analysis) of the compounds of training set results in the following correlation.
log(1/IC50)= -5.709(1.466) Ks +3.343(1.402) E3e +6.689(4.211) P2u +4.114(1.553)…… (1)
n = 30 r = 0.949 s = 0.212 F(3,26) = 77.906 (4.64)
r2cv= 0.858, r2pred = 0.622
The most significant parameters governing the potency of compounds were found to be Ks (K global shape Index/Weighted by related I-state), E3e (3rd component accessibility directional WHIM index/weighted by relative Sanderson electro negativities), P2u (2nd component shape directional WHIM index/Unweighted) with no remarkable mutual correlation between them as shown in Table 3. In (1) IC50 is the molar concentration of the compound leading to 50% inhibition of enzyme. Whereas, n is the number of data points, ‘r’ is the correlation coefficient, s is the standard deviation and F refers to the Fischer ratio between the difference of observed and calculated activities and the parentheses data with ‘+’/‘-’ sign are 95% confidence intervals[22]. The value within the parentheses for F is the standard F-value at 99% level. The correlation expressed by (1) seems to be highly remarkable and its internal and external validation can be judged by r2cv and r2pred values that are 0.858 and 0.622 respectively. The r2cv was calculated by using the formula as given in (2).
r2cv = 1 − [Σi (yi,obsd − yi,pred )2 / Σi (yi,obsd – yav,obsd )2 ] ……(2)
where, yi,obsd and yi,pred are the observed and predicted(from LOO) activity values of compound, respectively, and yav,obsd is the average of the observed activities of all compounds used in the correlation [23]. The correlation is assumed to be valid if r2cv> 0.60. From this belief, the correlation expressed by (1) seems to be quite licit. However, the model’s predictive capability is judged by predicting the biological activity of the compounds [24] in the test set using it and calculating the value of r2pred, which is defined as follows:
r2pred = 1 − [Σi(yi,obsd − yi,pred)2 / Σi(yi,obsd − yav,obsd )2 ] ……..(3)
where, yi,obsd and yi,pred are referred to the observed and the predicted biological activity values of compounds in the test set and yi,obsd is same as in (2) [25]. The biological activity values predicted from this equation for the test set compounds are given in Table 1 [26]. A comparative analysis shows that the predicted values are in very good concurrence with the corresponding observed ones. Same as in training set calculated values were found to be in excellent agreement with the observed ones. QSAR studies has not been performed earlier on the series of chiral tetrahydronaphthalene-fused spirooxindole. 3D-QSAR studies was performed on series of indenopyrazole inhibitors [27], found that cross-validated product (r2cv) was 0.845 and 0.763 for COMFA and COMSIA respectively, while 3D-QSAR studies of Azolium analogues, showing r2cv of 0.790 [28]. As compared to our present studies, r2cv is 0.858, showing better predictive ability of the model.All these observations can be better visualized in the graphs drawn between the observed and predicted activities of the compounds for both the sets (Figure 4). We have predicted some new compounds (Table 2) on the basis of (1).The structure of predicted compounds was modified and the variables were applied in (1) to calculate their activity. The compounds from serial no. 1 to serial no. 10 in Table 2 are showing higher activity from the base series (Table 1).
Docking results
Computer aided drug discovery has become a crucial methodology to perform various techniques such as hit identification, lead optimization and structure based virtual screening [29-31]. Docking is the prophecy of ligand conformation and posing within a targeted binding site. Docking studies focuses on accurate structural modelling and correct activity prediction [32-35]. By using Mole Gro Virtual Docker validation of the protein was done where the protein gets validated by Iterated Simplex (Plant Score Grid) Algorithm with RMSD Score 0.3569 and all the predicted compounds were docked in protein molecule (PDB ID 2W9Z). Docking results are mentioned in Table 4 in comparison with four other drugs which are already available in market namely as Camptothecine, Etoposide, Paclitaxel and Vinblastine. When we compare the Docking results of compounds showing comparable results with the licensed compounds. Compound 10 (table 4)is having best interaction with the target protein among all the predicted compounds. The interaction of the predicted compounds is almost with the same residues as the interaction shown by the marketized drugs, i.e. with the Arg(153), Ala(154), Tyr(183), Arg(172), Gly(170) and Thr(168) showing the same course of binding just like commercial ones.
Ks | E3e | P2u | |
---|---|---|---|
Ks | 1.000 | 0.012 | 0.563 |
E3e | 1.000 | -0.293 | |
P2u | 1.000 |
Compound | Total interaction energy | H-bond energy | No. of H bonds | H bonds | H bond length (Å) | Mole dock score | Internal pose |
---|---|---|---|---|---|---|---|
1 | -94.944 | -6.45839 | 4 | N(0)-Ala(154) N(0)-Arg(153) O(7)-Arg(172) O(7)-Arg(172) |
2.86 3.22 3.27 2.60 |
-94.84 | 0.10645 |
2 | -101.668 | -5.64745 | 4 | O(20)-Gly(150) O(20)-Arg(153) O(7)-Arg(172) N(0)-Ala(154) |
2.59 3.54 2.90 2.75 |
-98.82 | 2.84939 |
3 | -98.2637 | -4.54535 | 4 | N(0)-Ala(154) N(0)-Arg(153) O(7)-Arg(172) O(7)-Arg(172) |
2.89 3.40 2.64 3.51 |
-94.55 | 3.71195 |
4 | -94.0225 | -4.94002 | 4 | O(7)-Arg(172) O(7)-Arg(172) N(0)-Ala(154) N(0)-Arg(153) |
3.49 2.66 2.78 3.35 |
-94.59 | -0.56595 |
5 | -90.994 | -1.25942 | 2 | O(7)-Arg(172) O(7)-Arg(172) |
2.86 3.47 |
-88.50 | 2.48961 |
6 | -112.297 | -1.64759 | 2 | O(7)-Arg(172) O(10)-Arg(172) |
2.56 3.21 |
-106.38 | 5.91887 |
7 | -118.202 | -3.97304 | 4 | O(20)-Arg(129) O(7)-Arg(172) O(10)-Arg(172) N(22)-Arg(172) |
2.60 2.54 3.29 3.53 |
-112.96 | 5.23814 |
8 | -105.326 | -7.85012 | 4 | S(16)-Ala(154) S(16)-Tyr(183) O(10)-Gly(150) N(22)-Arg(153) |
2.80 2.60 3.44 3.19 |
-106.69 | -1.35987 |
9 | -95.1054 | -6.65452 | 4 | N(0)-Ala(154) N(0)-Arg(153) O(7)-Arg(172) O(7)-Arg(172) |
2.86 3.23 3.22 2.59 |
-95.18 | -0.07477 |
10 | -95.6396 | -9.46884 | 6 | N(0)-Ala(154) N(0)-Arg(153) O(25)-Asp(130) O(25)-Thr(168) O(7)-Arg(172) O(7)-Arg(172) |
2.89 3.16 3.01 2.67 2.56 3.40 |
-94.5266 | 1.11296 |
Camptothecine | -124.985 | 0 | NIL | NA | NA | -107.798 | 17.1868 |
Etoposide | -147.611 | -17.304 | 10 | O(35)-Ile(176) O(34)-Arg(172) O(22)-Tyr(183) O(41)-Arg(153) O(41)-Ala(154) O(41)-Gly(150) O(42)-Thr(17) O(1)-Tyr(162) O(31)-Tyr(162) O(31)-Thr(168) |
3.28 2.94 2.71 2.60 3.43 2.59 3.39 3.08 3.32 2.49 |
-137.537 | 10.0735 |
Paclitaxel | -170.871 | -6.69016 | 8 | O(53)-Arg(153) O(51)-Arg(153) O(29)-Tyr(162) O(29)-Thr(168) N(30)-Thr(168) N(30)-Tyr(162) O(24)-Arg(172) O(28)-Arg(172) |
3.46 2.74 3.19 3.17 2.99 3.47 3.32 3.47 |
-154.153 | 16.7174 |
vinblastine | -196.239 | -1.56044 | 5 | O(33)-Lys(36) O(33)-Asp(148) O(33)-Phe(149) N(35)-Asp(148) N(35)-Lys(36) |
2.87 2.93 3.54 2.95 2.02 |
-187.975 | 8.26375 |
ADME Results
Water Solubility
Having a soluble molecule smoothens the handling and formulation of many drugs [36]. In oral administration of drug solubility plays a major role by influencing its absorption rate [37]. In Swiss ADME water solubility of the compounds were predicted on the basis of two topological methods ESOL method [38] and second one is the method that was adapted by Ali et al. [39]. All the values that were predicted are decimal logarithm of the molar solubility in water and it also provides the solubility in mol/l and mg/ml [40] as shown in Table 5. Predicted compounds shows better solubility properties then Vinblastine and Paclitaxel, among which compound 2, 7 and 10 shows high solubility, this depicts that the predicted drugs are having higher propensity to dissolve in the aqueous media so as the drug molecule can be successfully absorbed by the intestine and administered.
S.No. | logS (ESOL) |
Solubil-ity | Class | logS (Ali) |
Solubility | Class | logS (SILICOS-IT) |
Solubility | Class |
---|---|---|---|---|---|---|---|---|---|
1 | -4.20 | 2.93E-02mg/ml; 6.31e-05 mol/l | Moderately Soluble | -4.19 | 3.03e-02 mg/ml; 6.53e-05 mol/l |
Moderately Soluble | -6.55 | 1.32e-04mg/ml; 2.84e-07 mol/l |
Poorly Soluble |
2 | -3.28 | 2.34e-01mg/ml; 5.26e-04 mol/l |
Soluble | -3.28 | 2.33e-01 mg/ml; 5.23e-04 mol/l |
Soluble | -5.38 | 1.88e-03 mg/ml; 4.22e-06 Mol/l |
Moderately Soluble |
3 | -3.94 | 5.14e-02 mg/ml; 1.15e-04 mol/l |
Soluble | -3.93 | 5.31e-02 mg/ml; 1.19e-04 mol/l |
Soluble | -6.23 | 2.65e-04 mg/ml; 5.92e-07 mol/l |
Poorly Soluble |
4 | -3.61 | 1.15e-01 mg/ml; 2.48e-04 mol/l |
Soluble | -3.52 | 1.41e-01 mg/ml; 3.05e-04 mol/l |
Soluble | -6.04 | 4.17e-04 mg/ml; 9.04e-07 mol/l |
Poorly Soluble |
5 | -3.95 | 5.01e-02 mg/ml; 1.12e-04 mol/l |
Soluble | -3.68 | 9.44e-02 mg/ml; 2.11e-04 mol/l |
Soluble | -6.42 | 1.71e-04 mg/ml; 3.82e-07 mol/l |
Poorly Soluble |
6 | -4.29 | 2.24e-02 mg/ml; 5.18e-05 mol/l |
Moderately Soluble | -4.08 | 3.56e-02 mg/ml; 8.22e-05 mol/l |
Moderately Soluble | -6.60 | 1.08e-04 mg/ml; 2.51e-07 mol/l |
Poorly Soluble |
7 | -3.62 | 1.02e-01 mg/ml; 2.37e-04 mol/l | Soluble | -3.44 | 1.56e-01 mg/ml; 3.62e-04 mol/l |
Soluble | -5.75 | 7.68e-04 mg/ml; 1.78e-06 mol/l |
Moderately Soluble |
8 | -4.55 | 1.28e-02mg/ml; 2.85e-05 mol/l |
Moderately Soluble | -4.34 | 2.03e-02 mg/ml; 4.52e-05 mol/l |
Moderately Soluble | -6.92 | 5.38e-05 mg/ml; 1.20e-07 mol/l |
Poorly Soluble |
9 | -3.61 | 1.15e-01 mg/ml; 2.48e-04 mol/l |
Soluble | -3.52 | 1.41e-01 mg/ml; 3.05e-04 mol/l |
Soluble | -6.04 | 4.17e-04 mg/ml; 9.04e-07 mol/l |
Poorly Soluble |
10 | -3.28 | 2.34e-01 mg/ml; 5.26e-04 mol/l |
Soluble | -3.28 | 2.33e-01 mg/ml; 5.23e-04 mol/l |
Soluble | -5.38 | 1.88e-03 mg/ml; 4.22e-06 mol/l |
Moderately Soluble |
Vinblastine | -6.84 | 1.17e-04 mg/ml; 1.44e-07 mol/l |
Poorly Soluble | -6.81 | 1.25e-04 mg/ml; 1.54e-07 mol/l |
Poorly Soluble | -8.46 | 2.80e-06 mg/ml; 3.45e-09 mol/l |
Poorly Soluble |
Paclitaxel | -6.66 | 1.85e-04 mg/ml; 2.16e-07 mol/l |
Poorly Soluble | -8.00 | 8.61e-06 mg/ml; 1.01e-08 mol/l |
Poorly Soluble | -8.00 | 1.34e-06 mg/ml; 1.57e-09 mol/l |
Poorly Soluble |
Camptothecin | -3.49 | 1.14e-01 mg/ml; 3.27e-04 mol/l |
Soluble | -3.07 | 2.99e-01 mg/ml; 8.58e-04 mol/l |
Soluble | -5.83 | 5.20e-04 mg/ml; 1.49e-06 mol/l |
Soluble |
Etoposide | -3.75 | 1.05e-01 mg/ml; 1.78e-04 mol/l |
Soluble | -3.55 | 1.65e-01 mg/ml; 2.81e-04 mol/l |
Soluble | -3.18 | 3.85e-01 mg/ml; 6.55e-04 mol/l |
Soluble |
Pharmacokinetics
In this section ADME behavior of the compounds were investigated on the basis of various models. Skin permeability coefficient (Kp) were predicted by using Multiple linear regression that was adapted by Potts and Guy [41] who found the linear correlation between Kpand its molecular size and lipophilicity. Passive human gastrointestinal absorption (HIA) and Blood-brain barrier (BBB) permeation both were predicted on the basis of BOILED-Egg model [42]. P-gp plays an important role in protecting Central Nervous System (CNS) from Xenobiotics [43]. Cytochromes P450 (CYP) interaction with the molecule plays key role in drug elimination through metabolic biotransformation [44]. 50-90% of estimated therapeutic molecules are substrate of 5 major isoforms (CYP1A2, CYP2C19, CYP2C9, CYP2D6, CYP3A4) [45,46]. Inhibition of these isoenzymes is one of the major causes of pharmacokinetics-related drug-drug interactions [47, 48]. The results of pharmacokinetic properties of the predicted compounds along with marketized drugs are cited in table 6. Intestinal absorption of proposed compounds is higher while of commercial once are not except Camptothecin. All the proposed compounds and licensed compounds are acting as substrate for P-gp, not penetrating Blood-Brain barrier, not inhibiting CYP2C19, CYP1A2 and CYP2C9 except Camptothecin, as it showing the inhibition of CYP1A2 and CYP2C9. All the proposed compounds and Etoposide are inhibiting CYP2D6 while other are not. Compound 6, 8, Camptothecin and Vinblastine are inhibiting CYP3A4 while non other is inhibiting. All the proposed one are having comparable skin permeability with the licensed one.
GI Absorption | BBB Permeant |
P-gp Substrate |
CYP1A2 inhibitor |
CYP2C19 inhibitor |
CYP2C9 inhibitor |
CYP2D6 inhibitor |
CYP3A4 Inhibitor |
log Kp (Skin permeation) (in cm/s) |
|
---|---|---|---|---|---|---|---|---|---|
1 | High | No | Yes | No | No | No | Yes | No | -7.65 |
2 | High | No | Yes | No | No | No | Yes | No | -8.45 |
3 | High | No | Yes | No | No | No | Yes | No | -7.73 |
4 | High | No | Yes | No | No | No | Yes | No | -8.29 |
5 | High | No | Yes | No | No | No | Yes | No | -7.71 |
6 | High | No | Yes | No | No | No | Yes | Yes | -7.15 |
7 | High | No | Yes | No | No | No | Yes | No | -7.87 |
8 | High | No | Yes | No | No | No | Yes | Yes | -7.07 |
9 | High | No | Yes | No | No | No | Yes | No | -8.29 |
10 | High | No | Yes | No | No | No | Yes | No | -8.45 |
Camptothecin | High | No | Yes | Yes | No | Yes | No | Yes | -7.19 |
Etoposide | Low | No | Yes | No | No | No | Yes | No | -9.46 |
Paclitaxel | Low | No | Yes | No | No | No | No | No | -8.91 |
Vinblastine | Low | No | Yes | No | No | No | No | Yes | -8.49 |
Medicinal Chemistry
To identify potentially problematic fragments, two complimentary pattern recognition methods were used. PAINS are molecules that contains substructures which shows potent response in assays irrespective of the protein target. Leads are subjected to chemical modifications that will probably increase size and lipophilicity [49]. So, the leads should be smaller and less hydrophobic than drug-like molecules. Synthetic accessibility ranges from 1(very easy) to 10(very difficult). It helps in the selection of most promising virtual molecules that will be synthetized. The proposed compounds are easily synthesized ac compared to the licesenced compounds as they are showing the synthetic accessibility between 4.77 to 5.20, while commercial compounds are having the synthetic accessibility of 6.27 (Etoposide), 8.34 (Paclitaxel), 9.69 (Vinblastine). Only Camptothecin is easily synthesized as compared to all compounds with synthetic accessibility of 3.84.Camptothecin is obeying the rules of PAINS, Brenk and Lead likeness while other compounds are showing violations of Brenk and Leadlikeness.(Tables 7 & 8).
Pains | Brenk | Leadlikeness | Synthetic Accessibility | |
---|---|---|---|---|
1 | 0 alert | 3 alerts: alkyl_halide, aniline, thiol_2 | No; 1 violation: MW>350 | 4.88 |
2 | 0 alert | 3 alerts: alkyl_halide, aniline, thiol_2 | No; 1 violation: MW>350 | 4.90 |
3 | 0 alert | 3 alerts: alkyl_halide, aniline, thiol_2 | No; 1 violation: MW>350 | 4.95 |
4 | 0 alert | 4 alerts: alkyl_halide, aniline, phosphor, thiol_2 | No; 1 violation: MW>350 | 5.20 |
5 | 0 alert | 3 alerts: alkyl_halide, phosphor, thiol_2 | No; 1 violation: MW>350 | 5.08 |
6 | 0 alert | 2 alerts: alkyl_halide, thiol_2 | No; 1 violation: MW>350 | 4.82 |
7 | 0 alert | 2 alerts: alkyl_halide, thiol_2 | No; 1 violation: MW>350 | 4.77 |
8 | 0 alert | 2 alerts: alkyl_halide, thiol_2 | No; 1 violation: MW>350 | 4.75 |
9 | 0 alert | 4 alerts: alkyl_halide, aniline, phosphor, thiol_2 | No; 1 violation: MW>350 | 5.07 |
10 | 0 alert | 3 alerts: alkyl_halide, aniline, thiol_2 | No; 1 violation: MW>350 | 4.93 |
Camptothecin | 0 alert | 0 alert | Yes | 3.84 |
Etoposide | 0 alert | 0 alert | No; 1 violation: MW>350 | 6.27 |
Paclitaxel | 0 alert | 2 alerts: isolated_alkene, more_than_2_esters | No; 3 violations: MW>350, Rotors>7, XLOGP3>3.5 | 8.34 |
Vinblastine | 0 alert | 2 alerts: isolated_alkene, more_than_2_esters | No; 3 violations: MW>350, Rotors>7, XLOGP3>3.5 | 9.69 |
log Po/w (iLOGP) | log Po/w (XLOGP3) | log Po/w (WLOGP) | log Po/w (MLOGP) | log Po/w (SILICOS-IT) | Consensus Log Po/w | |
---|---|---|---|---|---|---|
1 | 2.49 | 2.09 | 2.09 | 2.90 | 3.08 | 2.53 |
2 | 1.95 | 0.81 | 0.84 | 1.88 | 1.96 | 1.49 |
3 | 2.31 | 1.84 | 2.24 | 2.80 | 2.86 | 2.41 |
4 | 2.50 | 1.17 | 1.72 | 2.69 | 2.22 | 2.06 |
5 | 2.84 | 1.85 | 2.13 | 3.25 | 2.94 | 2.60 |
6 | 2.60 | 2.52 | 2.65 | 3.36 | 3.58 | 2.94 |
7 | 2.60 | 1.49 | 1.25 | 2.43 | 2.68 | 2.09 |
8 | 2.74 | 2.77 | 2.50 | 3.47 | 3.80 | 3.05 |
9 | 2.49 | 1.17 | 1.72 | 2.69 | 2.22 | 2.06 |
10 | 1.83 | 0.81 | 0.84 | 1.88 | 1.96 | 1.47 |
Camptothecin | 2.49 | 1.74 | 1.82 | 1.64 | 3.29 | 2.20 |
Etoposide | 3.24 | 0.60 | 1.01 | -0.14 | 0.95 | 1.13 |
Paclitaxel | 3.60 | 3.66 | 3.41 | 1.70 | 4.59 | 3.39 |
Vinblastine | 4.99 | 3.88 | 2.85 | 2.35 | 4.72 | 3.76 |
Drug Likeness
All the predicted compounds obey Lipinski Rule with 0 violations whereas Etoposide, Paclitaxel and Vinblastine shows multiple violations. Compound 1, 3, 5, 6, 7,8 and Camptothecinobeys Ghose, Veber, Egan and Muegge rules as well (Table 9). The Bioavailability score of the proposed compounds are higher i.e. 0.55 as compared to the licensed compounds as they are showing very less bioavailability score i.e. 0.17 except Camptothecin (bioavailability score = 0.55) this means that our proposed molecules can enter the systemic circulation at a much rate and can act much earlier as compared to commercial once.
Lipinski | Ghose | Veber | Egan | Muegge | Bioavailability Score | |
---|---|---|---|---|---|---|
1 | Yes | Yes | Yes | Yes | Yes | 0.55 |
2 | Yes | Yes | Yes | No; 1 violation: TPSA>131.6 | Yes | 0.55 |
3 | Yes | Yes | Yes | Yes | Yes | 0.55 |
4 | Yes | No; 1 violation: MR>130 | Yes | Yes | Yes | 0.55 |
5 | Yes | Yes | Yes | Yes | Yes | 0.55 |
6 | Yes | Yes | Yes | Yes | Yes | 0.55 |
7 | Yes | Yes | Yes | Yes | Yes | 0.55 |
8 | Yes | Yes | Yes | Yes | Yes | 0.55 |
9 | Yes | No; 1 violation: MR>130 |
Yes | Yes | Yes | 0.55 |
10 | Yes | Yes | Yes | No; 1 violation: TPSA>131.6 | Yes | 0.55 |
Camptothecin | Yes | Yes | Yes | Yes | Yes | 0.55 |
Etoposide | No; 2 violation: MW>500, NorO>10 | No; 3 violation: MW>480, MR>130, #atoms>70 | No; 1 violation: TPSA>140 | No; 1 violation: TPSA>131.6 | No; 2 violation: TPSA>150, H-acc>10 | 0.17 |
Paclitaxel | No; 2 violation: MW>500, NorO>10 | No; 3 violation: MW>480, MR>130, #atoms>70 |
No; 2 violation: Rotors>10, TPSA>140 |
No; 1 violation: TPSA>131.6 | No; 3 violation: MW>600, TPSA>150, H-acc>10 | 0.17 |
Vinblastine | No; 2 violation: MW>500, NorO>10 | No; 3 violation: MW>480, MR>130, #atoms>70 |
No; 1 violation: TPSA>140 | No; 1 violation: TPSA>131.6 | No; 4 violation: MW>600, TPSA>150, #rings>7, H-acc>10 | 0.17 |
Toxicity
It’s important to indicate the toxic potential of the compounds [50]. In- silico toxicity is developing as an integral platform as it prevents plants, animals, humans and environment that could be harmed due to the toxicity of predicted compounds [51]. The toxicity of the predicted compounds was checked by using the Pro Tox II and admetsar 2 web servers along with the drugs that are already available in market as shown in table 10. Drug Hepatotoxicity is one of the major reasons of withdrawing drug form the market as it causes acute live failure [52]. Data used for the prediction of carcinogenicity are collected from Carcinogenic Potency Database (CPDB) and CEBS database [53,54] that gives 85% accurate results on external validation. Mutagens (chemicals that cause mutations in the DNA of the cell [55] are responsible for the mutagenicity and can cause cancer. So, the compounds were predicted, considering that the toxicity of the compounds should be low. Predicted compounds are least or non-toxic i.e. on various parameters like Hepatotoxicity, Carcinogencity, Immunotoxicity, Mutagenecity, Cytotoxicity, heat shock factor response elelment (HSE) and Phosphoprotein (Tumor Suppressor) p53. On the other hand, drugs available in market, all of them are immunotoxic. Camptothecin, Paclitaxel and Vinblastine are cytotoxicity. Paclitaxel, Camptothecin, Etoposide is showing Phosphoprotein (Tumor Suppressor) p53 and Etoposide is having heat shock factor response elelment (HSE) as shown in Table 10.
Hepatotoxicity | Carcinogencity | Immunotoxicity | Mutagenecity | Cytotoxicity | Heat shock factor response elelment(HSE) | Phosphoprotein(Tumor Suppressor) p53 | |
---|---|---|---|---|---|---|---|
1 | Inactive | Inactive | Inactive | Inactive | Inactive | Inactive | Inactive |
2 | Inactive | Inactive | Inactive | Inactive | Inactive | Inactive | Inactive |
3 | Inactive | Inactive | Inactive | Inactive | Inactive | Inactive | Inactive |
4 | Inactive | Inactive | Inactive | Inactive | Inactive | Inactive | Inactive |
5 | Inactive | Inactive | Inactive | Inactive | Inactive | Inactive | Inactive |
6 | Inactive | Inactive | Inactive | Inactive | Inactive | Inactive | Inactive |
7 | Inactive | Inactive | Inactive | Inactive | Inactive | Inactive | Inactive |
8 | Inactive | Inactive | Inactive | Inactive | Inactive | Inactive | Inactive |
9 | Inactive | Inactive | Inactive | Inactive | Inactive | Inactive | Inactive |
10 | Inactive | Inactive | Inactive | Inactive | Inactive | Inactive | Inactive |
Camptothecin | Inactive | Inactive | Active | Inactive | Active | Inactive | Active |
Etoposide | Inactive | Inactive | Active | Inactive | Inactive | Active | Active |
Paclitaxel | Inactive | Inactive | Active | Inactive | Active | Inactive | Active |
Vinblastine | Inactive | Inactive | Active | Inactive | Active | Inactive | Inactive |
Conclusion
A series of 10 new compounds of THN-fused spirooxindole derivatives is proposed on the basis of our QSAR models that possess higher potency. Docking results shows that these compounds have better binding affinity than the marketed compounds. ADME properties are showing that the proposed compounds are better lead like molecules as compared to the licensed compounds. Toxicity prediction ensures that these compounds are least or non-toxic as compared to licensed compounds available. Therefore, these compounds can be synthesized and tested in the wetlab.
Conflict of Interests
The author declared that, having no conflict of interests.
Acknowledgements
The authors are thankful to the Meerut Institute of Engineering and Technology for providing the infrastructure and support for completion of this project. This work was also supported by the Molexus. Our profound gratitude is expressed to Mr. René Thomsen for providing the academic License of MVD software used for Docking. We acknowledge Chem Axon Ltd. (www.chemaxon.com) for the academic license agreement. ADMET properties were studied by the help of Swiss ADME and Pro Tox-ll.
References
- Baker SJ and Reddy EP. Genes & Cancer, 2012. 3: p. 658–669.
- Ciarallo S, Subramaniam V, Hung W et al., Mol Cell Biol., 2002. 22: p. 2993-3002.
- Bockstaele L, Kooken H, Libert F et al., Mol Cell Biol., 2006. 26: p. 5070-85.
- El-Deiry WS, Tokino T, Velculescu VE et al., Cell, 1993. 75: p. 817–825.
- Waldman T, Kinzler KW and Vogelstein B. Cancer Res., 1995. 55: p. 5187–5190.
- An HX, W M and Beckmann G. et al., Am J Pathol., 1999. 154: p. 113–118.
- Bianchi AB, Fischer SM, Robles AI et al., Oncogene, 1993. 8: p. 1127–1133.
- Iida H, Towatari M, Tanimoto M et al., Blood, 1997. 90: p. 3707–3713.
- Lantsov D, Meirmanov S, Nakashima M et al., Histopathology, 2005. 47: p. 248–256.
- Marone M, Scambia G, Giannitelli C et al., Int J Cancer, 1998. 75: p. 34–39.
- Kato A, Takahashi H, Takahashi Y et al., J Biol Chem., 1997. 272: p. 8065-70.
- Baker SJ andReddy PE. Genes & Cancer, 2012. 3(12): p. 658–669.
- Kaur M, Singh M, Chadha N et al., Eur J Med Chem., 2016. 123: p. 858–894.
- Li-Ming Z, Ren-Yu Q and Yang GF, Expert Opin DrugDiscov., 2020. 15(5): p. 603-625
- Huang L, Zhang XY, Li J et al., Eur J Org Chem, 2014, 2: p. 338–349.
- Peng LJ, Zhou G and Han SN. Chin J Synth Chem, 2016. 24: p. 669–672.
- Natarajan A, Almansour AI, Raju S et al., Tetrahedron, 2018. 74: p. 5358–5366.
- Shyamsivappan S, Vivek R, Saravanan A et al., Medchemcomm. 2019. 10: p. 439–449.
- Wanga B, Pengb Fu, Huanga W et al., Acta Pharmaceutica Sinica B, 2019. 12: p. 2211-3835.
- Perkins R, Hong. Fang, Weida et al., Environmental Toxicology and Chemistry, 2003. 8: p. 1666–1679.
- Bradbury S, Toxicology Letters, 1995. 79: p. 229–237.
- Drwal MN, Banerjee P, Dunkel M et al., Nucleic acids research, 2014. 42: p. W53-W58.
- Jaeschke H, Gores GJ, Cederbaum AI et al., 2002. 65(2): p. 166-176.
- Kar S and Roy, Ind J Biochem Biophy, 2011. 48(2): p. 111-22.
- Descotes J, Immunotoxicity of drugs and chemicals, 1986.
- Agarwal N, Bajpai A, Srivastava V et al., Structural Biology, 2013. 810691: p. 11.
- Muzaffar-ur-RehmanMd, Gunda SK, Corrie L et al., Indo American J Pharm Sci, 2017. 4(9): p. 2981-2993.
- Rondla R, Rao LSP, Ramatenki V et al., J Mol Struct, 2017.1134: p. 482-491.
- Bajorath J, Nat Rev Drug Discov., 2002. 1: p. 882–894.
- Walters WP, Stahl MT and Murcko MA, Drug Discov. Today, 1998. 3: p. 160–178.
- Langer Tand Hoffmann RD, Curr Pharm Design, 2001. 7: p. 509–527.
- Kitchen D, Decornez H and Furr JR. Nat Rev Drug Discov, 2004. 3: p. 935–949.
- ADMET structure-activity relationship server. http://lmmd.ecust.edu.cn/admetsar2/
- ADME and drug likeness properties evaluation server. http://www.swissadme.ch/index.php
- Toxicity prediction server.http://tox.charite.de/protox_II/index.php?site=compound_search_similarity
- TJ Ritchie, Macdonald SJF, Peace S et al., Med Chem Commun., 2013. 4: p. 673.
- Ottaviani G, Gosling DJ, Patissier C et al., Eur J Pharm Sci, 2010. 41: p. 452–457.
- Delaney JS, J Chem Inf Model., 2004. 44: p. 1000–1005.
- Ali J, Camilleri P, Brown MB et al., J Chem Inf Model., 2012. 52: p. 420–428.
- Daina A, Michielin O and Zoete V, Sci Rep, 2017. 7:p. 42717.
- Potts ROand Guy RH. Pharm Res, 1992: 9: p. 663–669.
- Daina A and Zoete V. Chem Med Chem, 2016. 11: p. 1117–1121.
- Szakács G, Váradi A, Ozvegy-Laczka C et al., Today, 2008. 13: p. 379–393.
- Testa B and Kraemer SD, Chem Biodivers., 2007.5(11): p. 2171-2336.
- Wolf CR, Smith G and Smith RL, Brit Med J, 2000. 320(7240): p. 987–990.
- Di L, Expert Opin Drug Metab Toxicol, 2014. 10: p. 379–393.
- Hollenberg PF, Drug Metab Rev., 2002, 34: p. 17–35.
- Huang SM, Strong JM, Zhang L et al., J Clin Pharmacol, 2008. 48: p. 662–670.
- Hann MM and Keserű GM, Nat Rev. Drug Discov., 2012. 11: p. 355–365.
- Wang Y, Xing J, Xu Y et al., Rev. Biophys., 2015. 48: p. 488–515.
- Raies AB and Bajic VB. Wiley Interdiscip. Rev. Comput. Mol. Sci., 2016. 6: p. 147–172.
- Siramshetty VB, Nickel J, Omieczynski C et al., Nucleic Acids Res., 2016. 44: p. D1080–D1086.
- Fitzpatrick RB, Med Ref Serv Q., 2008. 27: p. 303–311.
- Lea IA, Gong H, Paleja A et al., J Fostel Nucleic Acids Res., 2017. 45: p. D964–D971.
- Ames BN, Durston WE, Amasaki EY et al., Proc Natl Acad Sci. 1973. 70: p. 2281–2285.
Select your language of interest to view the total content in your interested language
Google Scholar citation report
Citations : 15261
Der Pharma Chemica received 15261 citations as per Google Scholar report
Der Pharma Chemica peer review process verified at publons
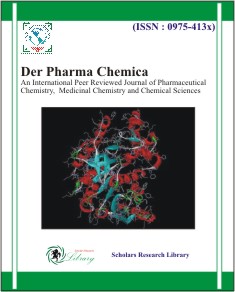